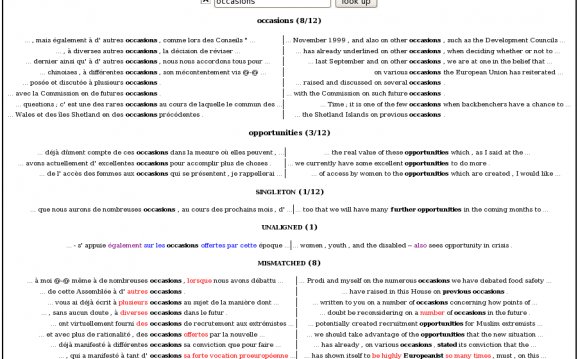
The first of twelve TAUS Open Source Machine Translation Showcases planned for the next three years took place over the weekend in Monaco just ahead of GALA’s annual conference. These free sessions are funded by the EC as part of the MosesCore project.
Here’s a short summary of the session with links to all presentations.
People have hoped to tear down language barriers and some have even made disastrously wrong five-year forecasts about what MT will be capable of ever-since the famous IBM-Georgetown experiments of 1954. The reality is that there will be continuous and gradual quality improvements in the next 5-10 years, but many frailties will remain.
However, there is latent demand and the language industry is now warming to the technology. We’ve moved from a handful of commercially usable MT providers to a few dozen in a few short years.
Many of these newcomers are using the open source statistical MT solution, Moses.
I suggested that there could potentially be 1000 providers as there must be at least this many translation companies making more than 1-million US dollars a year; i.e. you don’t need tremendously deep pockets to get going. The language combination, the nature of your client base and the training data you have available, will play heavily on your ROI calculations.
Moses uses the same data-driven technological approach as Google Translate and Microsoft Bing. However, it is not currently used for building general-purpose engines such as its bigger brothers. Instead it’s well suited to making specialized MT engines for specific clients and industry-domains, where it does better than its brethren.
There are a number of ways to adopt Moses. But in broad terms:
You can install, train and use your own engine. You might hire a consultant to help to get started and you may use additional open source components that aren’t provided as part of the Moses toolkit to help with installation and integration, inter alia. But ultimately, you own your engine, your data stays yours and you acquire real MT know-how.
Or you climb on the shoulders of those that have already been through the pain of bolting together everything that’s needed to include MT in a translation workflow. Those early mover language service providers and pure play MT specialists who fill Moses’ natural language processing, engineering and usability gaps to provide convenience through their commercialized offerings largely aimed at serving translation companies. Talks from Diego Bartolome (Tauyou), Andrejs Vasiljevs (Tilde/LetsMT) and Jie Jiang (Applied Language Solutions) gave participants a good overview of some of these offerings:
It’s worth taking a look at these slides as they give you a good overview of workflows, as well as where these guys differentiate themselves.
Serge Gladkoff/Renat Bikmatov (Logrus International), Joël Sigling (AVB Translations) and Gustavo Lucardi (Trusted Translations) spoke about their experiences building Moses engines. These are translation companies looking to use MT to optimize their margins and grow relationships with end clients. Their slides are useful for understanding the decision-making factors for this approach in more detail and getting a good feel for how long it takes to get results. They also provide some insight into how their own engines perform against commercial offerings.
MORE TRANSLATION VIDEO
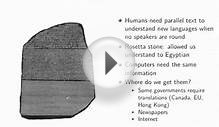
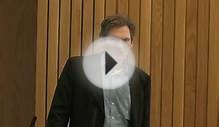
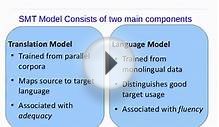